
The Impact of Process Modeling: Reducing Queue Times for COVID Testing
MoreSteam Client Services team member Dr. Lars Maaseidvaag has spent the last thirty years mastering and understanding the importance, value, and potential of virtual prototyping. Over the years, Lars has had the opportunity to use his expertise to work with clients across several industries, leveraging process modeling to design, redesign, and validate process changes.
Recently, he sat down and wrote his reflections on one impactful use of process modeling in the healthcare system.
The Case for Modeling
Those of you who know me or have read some of my past blogs know I have been on a thirty-year mission to bring dynamic process modeling to the continuous improvement world. That mission began when I was introduced to discrete event simulation modeling at The University of Texas in 1991.
The benefit of process modeling, as an extension to the traditional Lean Six Sigma toolkit, is that we can begin to understand process dynamics and interactions in a forward-looking manner, experimenting with what “could be” rather than just what “has been.” Bringing these tools to a wider audience was always a challenge, both in understanding the context of their use and learning the software tools available. As an industrial engineer myself, I’ve often used the phrase “by industrial engineers, for industrial engineers” to describe the available tools. They’re not cheap and tend to be complex to learn and use. Truth be told, the first version of MoreSteam’s Process Playground, designed and programmed by me, also fell into that category. Isn’t it perfectly clear that a negative batch size would mean a single part becomes many? Seemed like it to me.
That original Process Playground was introduced over ten years ago. About five years ago, a new version was created with a strong emphasis on the user experience, guided by MoreSteam’s EngineRoom product manager, Karina Dube. Karina’s expertise and education in designing high-quality user experiences led to a product that is simultaneously more powerful and easier to use. And there are no more negative batch sizes…
All of this self-promoting preamble has a purpose. When the pandemic began, rapid process design and prototyping quickly replaced continuous process improvement. Traditional Lean Six Sigma tools for process design are excellent tools, but there was no way to pilot concepts. We had to develop processes that had a high probability of working (and working well) the first time. “Live” pilots weren’t something organizations were willing to risk when we didn’t understand the spread of the virus and didn’t yet have many tools beyond physical distancing and masking to somewhat mitigate transmission. I worked with many clients, building rapid prototypes of various processes using process modeling. Without question, the most impactful of these I had the privilege of working on was with a world-renowned research hospital.
The Problem
The problem was relatively simple, but the solution required understanding how variation in different parts of the process would interact to impact process performance. The hospital had sent home all non-essential workers to work from home at the beginning of the pandemic. Anyone now directly involved in patient care began to work from home. This included everyone from the accountants to the research scientists normally working in a lab environment. After several months, the hospital decided to start bringing employees back into the workplace. This was before the vaccines were available, so there was a screening process to reduce the chance of an infectious person being around others.
The issue was a fairly straightforward balance problem (I’ve rounded the numbers, but the order of magnitude is correct): Figure out how to move a thousand people through a process that takes one to five minutes, with a fixed number of screening stations, very limited physical space, and the requirement of maintaining six-foot physical distancing.
Step One - Traditional Lean
The math is pretty simple. Ten screening stations at 2.5 minutes per person means that we can process 240 people per hour under perfect conditions. Now introduce a demand of 1000 people showing up at roughly the same time, and you have an average time-in-queue of 2 hours.
You read that right - the average time in queue was 2 hours per day! For 1000 people! The amount of lost productivity was staggering. And given that the process itself had room for about 25 people at a time, hundreds of people were left waiting outside in the parking lot, rain or shine.
The first step was to apply the lean method of Heijunka – level the load on a process by volume or type. In this case, we don’t have type variation, just a large volume of demand arriving at the same time. Applying Heijunka in the form of staggered start times allowed the arrival demand to spread over 5 hours, with a peak in hours 2 and 3 relative to hours 1, 4, and 5.
Leveling out demand to better match capacity was the first step, but the process itself was subject to a fair degree of variability. The process could be as quick as one minute if the computer questionnaire each employee had to answer and the temperature check didn’t flag the person for testing. However, if the person was flagged for testing, they entered the second part of the process, where they were swabbed and tested. There was randomness in the rate of those flagged for additional testing, randomness in the time to test, and, of course, randomness in the arrival of employees to the process. Under normal circumstances, we would take our spreadsheet-based Heijunka model and pilot the process, revising our staggered starts and process capacity as we learned from experience. The risk was too high, so we made the decision to build a simulation model to fine-tune the process before implementation.
Step Two - Process Modeling
The ultimate goal of the process model was to determine the best levels for the staggered arrival of demand and the staffing of the screening and testing processes. We did time studies to understand the task times associated with the screening and testing processes. That data, combined with the People-in-Process capacity dictated by the physical space that housed the process, gave us the capacity side of the equation.
We used triangular distributions to describe the screening and testing processes. We then modeled the highly variable arrival of employees to the process using an exponential distribution of the time between arrivals (a Poisson process for arrivals).
With the process model built, we conducted experiments to understand the interaction of the arrival variability with the process variability. The goal was to eliminate the unacceptably large queue times in the screening process as it was initially implemented before applying the Heijunka method or process modeling.
The Timeline and Results
Using the model, we developed a combination of staggered starts and a staffing plan for the ten screening stations that drove the average queue time to essentially zero. We eliminated hundreds of hours of lost productivity per day, and the risk of questionable physical distancing while waiting in queue disappeared.
This exercise is the very definition of “rapid” in rapid process prototyping. The initial screening process went live, and the giant queues formed. The senior leadership of the hospital demanded a solution immediately. My contact on the client side performed the time studies, and from the time I was contacted, it took just a few hours to build the model of the process. We met on a Zoom call to run the experiments a few days later. As the human resources staff pulled demand data, we were running experiments. Over a two-hour session, we refined the demand data, experimented with the model to determine the capacity side of the equation, and prepared a presentation for the board of directors showing that the process would be robust against the predicted variation and able to succeed in eliminating the long queues and lost time.
The process was approved and implemented, and the results were immediately apparent. Sometime later, I was talking to my client contact, and he said the board was ready to spend tens of thousands of dollars to create the extra capacity needed to solve the problem. Instead, we solved the problem with some Lean thinking and process modeling to ensure the Lean solution would stand up to the high degree of variation the process would encounter.
The board was ready to spend tens of thousands of dollars to create the extra capacity needed to solve the problem.
Throughout the beginning of the pandemic, I had many opportunities to contribute my modeling expertise to several client problems. Of them, all this effort, short and targeted as it was, was by far the most satisfying.
Over the last few years, I’ve seen a dramatic increase in clients adopting process modeling as a standard part of their Lean Six Sigma and continuous improvement toolkit. One client has even gone so far as to require a process model as part of the project discovery or measure phase. The concept is: If we can’t model it, then we probably don’t really understand it.
If you would like to learn more about process modeling, feel free to reach out to me at lars@moresteamclientservices.com. Or, feel free to read some of my past blogs or try out a free trial of EngineRoom, where Process Playground lives.
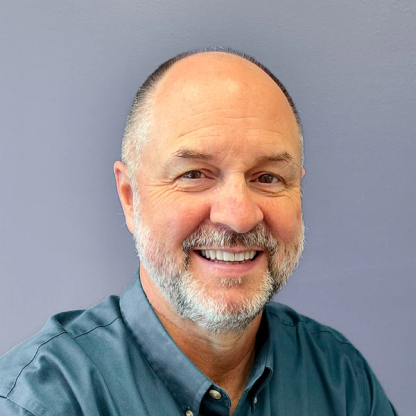
MoreSteam Client Services
Dr. Lars Maaseidvaag continues to expand the breadth and depth of the MoreSteam curriculum by integrating the learning of Lean tools and concepts with advanced process modeling methods. Lars led the development of Process Playground, a Web-based discrete event simulation program. Before coming to MoreSteam in 2009, he was the Curriculum Director for Accenture/George Group and has also worked in operations research and management consulting.
Lars received a PhD in Operations Research from the Illinois Institute of Technology. Prior to his PhD, Lars earned a M.S. in Operations Research and Industrial Engineering as well as an MBA from The University of Texas in Austin.
Use Cases for Process Modeling
- Examine more improvement alternatives than live pilots
- Fine-tune your solution before implementation
- Examine the impact of failure modes; perform a virtual FMEA
- Understand complex interactions within a process
- Optimize resource sharing across multiple value streams.
- A teaching tool to illustrate Lean Six Sigma concepts
Resources for Process Modeling
Help Center: What is Discrete Event Simulation (Process Modeling)?
Learn Process Playground: Kathy's Best Wursts Case Study
Recorded Webinar: Using Modeling to Teach Lean Six Sigma